Supply Chain Optimization
Cost to Serve Analysis – The Holy Grail of Sustainability
May 4, 2020
10 mins read

We breathe in the age of disruption. Technology is transforming supply chains across industries and its digital twin is a lot more powerful, flexible and adaptable. The goal of any tech implementation / association in the supply chain is very simple – facilitate customer demand fulfilment while remaining highly profitable by eliminating wastages. However, there are not a lot of tools currently which facilitate decision making in the supply chain. ERPs and its module systems handle and process unstructured data, which makes sense at an aggregated level, but when micro or entity level costs are to be understood, this information needs to be further processed.
For example, with the available unstructured data, can supply chain executives answer questions like: Are all products profitable, what is the cost of offering a product mix, what mix should be promoted? Impact of promised service levels (of different or a particular customer) on the transportation costs and eventually profitability and pricing strategy? More often than not, there is only intuition and no definite data-based analysis of these questions. The answers to these questions directly impact profitability, making the cost to serve analysis critical for an enterprise’s competitive edge.
According to the Logistics Bureau, most companies do not perform Cost to Serve Analysis. Following are the key inferences from their report:
- An average of 12% of the customers served, are actually loss bearing due to high distribution and storage costs
- 5-10 % of the product range made no contribution at all
- Up to 20% of customer orders made negative profit margins
So, what is a Cost to Serve study?

It’s a methodology which allows organisations to identify the total cost of servicing its customers at an individual customer and product level. The total cost here includes all the costs involved in a product’s value chain (Raw material to Customer door step). Business leaders can segregate and analyze individual customers or geographies and individual products or families or combinations of products and customers. This structured database can be used to identify supply chain opportunities like geographical expansion or cutting down on loss bearing products.
The next chronological question should be – how does one calculate the cost to serve? Manually tracing back a product from the customer doorstep to the raw material which comes from the supplier and everything in between might be more than a herculean task, as most costs are allocated as a fixed cost for a channel or a time period or a bulk of quantity. Advanced analytics coupled with Artificial intelligence has an answer to this problem. An activity-based modelling algorithm that can break down the entire supply chain into multiple activities, while calculating the costs at every activity, will serve the purpose.
Locus approach to Cost to Serve Analysis:
Locus consulting has formulated a 5-step framework (Figure 1) which enables enterprises to analyze various costs associated with the value chain journey of a product.
Org level stakeholders buy-in:
It is not surprising that internal agreement and buy-in from various stakeholders is the first step for the initiation of this study. A decision involving revenue impact, has to move across different tables in an organization. This primarily happens because there are multiple cost centers involved in a value chain and the P&L responsibility cannot be associated to a single department and looked at as a silo. For example, a decision involving a change in customer fulfillment strategy to reduce the cost of serving, has to be discussed with sales and marketing (For SLA impact), logistics (For cost impact), go-to-market strategy (For organisation strategy alignment) and warehousing teams (For resource planning and allocation), before the green light can be given.
Cost categorization at a function and process level:

Once the stakeholders are aligned to initiate the study, the next step would be to categorize the costs associated in a supply chain. Most supply chains have the following cost centers or functions: Procurement, Manufacturing, Warehousing, Logistics and Associated services. These functions in turn have multiple processes with costs linked to each of them. The reliability of any cost to serve analysis lies in capturing all the associated costs at a process level which can be aggregated back to a function level.
Across different customers, major cost variations can occur at a holding, distribution and customer support level, other parameters remain fairly stable. While across different products, variations can occur at any layer of the supply chain. Figure 2 presents a categorization (not exhaustive) that can be used as a good starting point to begin the study.
Cost breakdown at per unit of measurement level:
Most costs are normally recorded and defined at an aggregated level (like one month or one truck) which would have a fixed and/or a variable component. From a cost to serve perspective, the fixed component is hard to tackle as it represents a mix of products, channels, customers etc. Segregating these costs out for a single product or a single customer might not be manually possible because it becomes an intricate problem of segregation and the numbers to be crunched can be in the order of a few hundred megabytes. Hence, advanced analytics can be deployed to break down the cost at a product and at a customer level and unify it to a unit of measurement level. The unification process is absolutely essential, as it puts all the numbers on the same denomination for arithmetic operations.
For example, in a biofuel business, all the costs can be converted to a per gallon of fuel. Raw material required to produce a gallon of the fuel, manufacturing conversion cost associated with producing one gallon, transportation cost for moving one gallon from point A to point B. Obviously, there will be variations like different production processes, different modes of transportation, etc. at different legs of the supply chain, which the study should appropriately account for. These individual cost numbers can be used to calculate cost to serve and profit percentage realized by comparing with Sales orders or shipment history in the following manner:
Revenue – Quantity * (Cost of warehousing + Cost of transportation + cost of manufacturing + cost of raw materials + associated services cost + other costs) = Profit
The above formula is specific to serving a particular product to a particular customer.
Classification Matrices:
Insights from the cost to serve optimization study can be overwhelming, given the amount of data which has been turned into useful information. A systematic analysis approach is required to take informed decisions. This structure can be put across by charting out various variables in different quadrants confined by a characteristic. We have visualised the results in three different matrices arranged chronologically.
Customer classification matrix:
First up, is the customer classification matrix, decisions derived from this matrix can be immediately implemented.

The quadrants logically outline the characteristics and the action required by the organization to move towards profitability. Naturally, the best performing customers need to be retained with customer loyalty programs or a personal touch, while the worst performing customers need to be released from the system. The nature of the action to be taken will be unique to a sector or even unique to the organization based on their supply chain vision and organizational goals.
Product classification matrix:
Customer classification matrix provides no insights on the product’s profitability and will not enable any decision making with respect to product offering. It is vital to understand how the products offered are behaving with respect to its category or family or different categories and families, because offering a mix or different families always has a cost associated with it.

Naturally, the products with low demand and high profitability need to be invested upon to create the market or open a new channel to sell more along with an upgrade in product features/variants etc. While on the other hand, products with high demand and low profit need to undergo a change in strategy to cut down costs. This can include changes in the manufacturing process, change in pricing strategy or go-to-market strategy.
Product-Customer classification matrix:
An enterprise with few products but multiple customers might call out only for the customer classification matrix, while an organization with only a few big customers but with a lot of product variants might call for only the product classification matrix, but for all these scenarios, the study would be complete only if we can visualize the product and customer combination as a pair.

The Product-Customer classification matrix is a combination of the individual matrices, though not an immediate derivative. This matrix is also plotted from the results of the cost to serve study. Figure 5 demonstrates an example of the Product – Customer Classification Matrix. The bubbles depict the position of a particular Product – Customer (P – C) pair in the matrix. The size of all the bubbles are the same, as the magnitude is already a function of the X and Y coordinates.
Inferences derived from the previous charts can be validated in this matrix along with understanding changes in one entity with respect to the other. For example: consider a customer who has a low cost to serve in the customer classification matrix, because it is situated close to a warehouse or it orders bulk quantities which can be fulfilled by larger trucks. This should have a low cost to serve across different products as well. Customer C1 from figure 5 is a good candidate, as evident in P1C1, P2C1 and P3C1 combinations. In the same way, consider a product which has low cost to serve in the Product classification matrix, because it occupies less truck space or needs cheap raw materials to manufacture. This should have a low cost to serve across different customers as well. Product P2 from figure 5 is a good example as evident in P2C1 & P2C3 combinations.
There will always be cases where a product or customer overlaps with different variables, for example: P3 is a part of both high and low profits, this can be attributed to price variation or high distribution costs across different customer geographies. This matrix enables internal debates and discussions to understand the overlap behaviour, hence tapping out information confined to only one department earlier, which might not have been important enough to receive management’s attention. The overlap cases are particularly interesting as they do not have an immediate inference but require internal discussions to understand the reason for this behaviour, followed by steps to eradicate the higher costs.
Joint decision making for implementation:
Once the classification matrices are established, joint decisions should be taken to reduce the bottom line and strategize improvement in the top line. Visibility of relevant Cost to Serve data means organizations can make informed choices and better control their profitability instead of being forced to struggle with mediocre bottom line performance. Giving up revenue is no easy decision for any business, but it is a necessary decision to sustain in this dynamic market landscape.
Author – Amit Kumar Goenka, Associate Vice President, Consulting
Locus supply chain strategy consulting is committed to transforming supply chains from traditional cost centers to market differentiators. We help organizations optimize their supply chain and strategize sustained business growth through data-aided decision making. Locus consulting is the perfect fusion of supply chain expertise and cutting edge Artificial Intelligence capabilities to drive innovative and contextual solutions.
Related Tags:
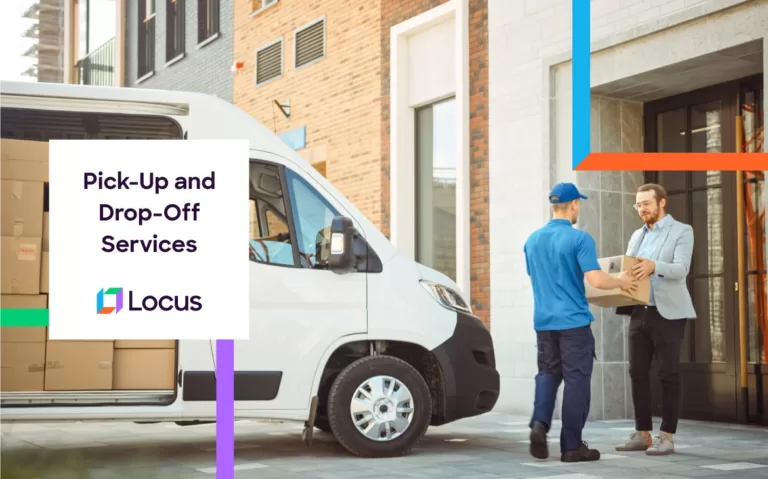
E-Commerce
Pick-Up and Drop-Off Services: How PUDOs Can Redefine Delivery Efficiency and Convenience?
Last Updated: Jan 2021 Pick-Up and Drop-Off Services: The biggest last-mile challenges facing the logistics industry are: failed deliveries and missed deliveries. Retail outlets have had a consistent collapse in in-store traffic since the outbreak of the COVID-19 pandemic. E-commerce has now become the preferred choice for any kind of purchase. The rise in home […]
Read more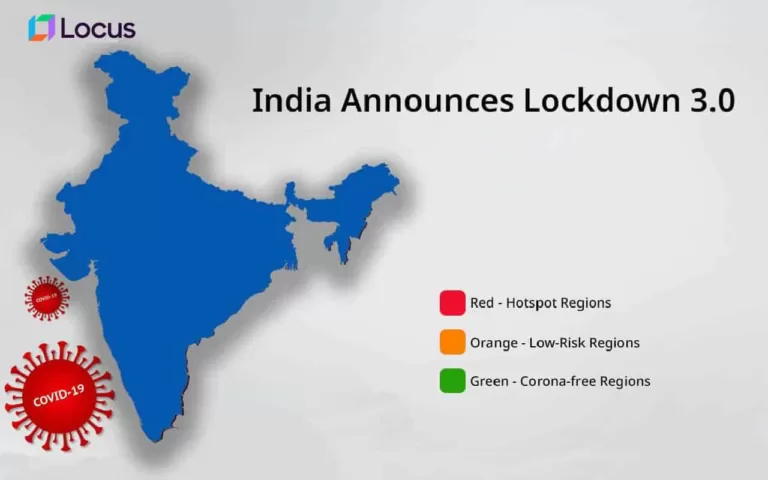
News
E-commerce Deliveries Resume in Green and Orange Zones
Ecommerce deliveries resume non-essentials in Green & Orange Zones. Check how efficient zone-based route planning for last-mile deliveries will be critical.
Read moreMOST POPULAR
EDITOR’S PICKS
SUBSCRIBE TO OUR NEWSLETTER
Stay up to date with the latest marketing, sales, and service tips and news
Cost to Serve Analysis – The Holy Grail of Sustainability